Main Research Topics
Temporal Networks:
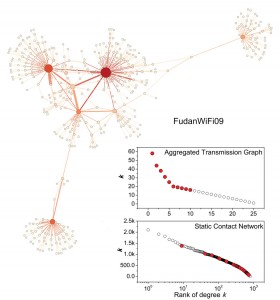
Small-degree individuals in the static contact network belong to several hubs (big-size vertices) in temporal network.
Since the discoveries of small-world and scale-free networks in the late of 1990s, we have witnessed fruitful and exciting advances to understand the hidden patterns behind complex connectivity features and characteristics of diverse large-scale networking systems. Extensive efforts and elegant attempts have been devoted to answering a fundamental question: how does the fascinating complex topological features affect or determine the collective behaviors and performance of the corresponding complex networked system. Temporal networks, where the edges are not continuously active but intermittent, fundamentally alter the transitivity of edges and induces that the temporal structure of edge activations can affect dynamics of systems interacting through the network, from disease contagion on the network of patients, information diffusion over an online social network to synchronizations on a coupled dynamic network. Especially, our group focus on how temporal characterizations of complex networks alter dynamics of epidemic processes on them, to help predict, forecast and control the epidemic spreading and information diffusion in online and offline populations.
Social Network Mining:
Understanding the universal characteristics of human mobility and physical interaction is crucial to reveal the complex dynamics of social system, and widely applied from prediction friendships to forecasting the spread of airborne pathogens and diffusion of information. With the development of smart portable devices and mobile communication, it is possible for us to collect human behavior traces as well as their collective interactions with high resolution. Taking advantage of these kind of data (e.g., FudanWiFi09 ), our research focuses on the empirical analysis of human traces in complex social system and tries to find out the co-occured diverse human mobility and interaction patterns. Strikingly, we study some neglected yet important phenomenons such as “familiar strangers” and time order of human behaviors which may be served as new views to better understand the impact of collective human behavior regularity on various diffusion/spreading dynamics.
Epidemic Dynamics:
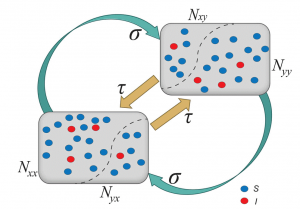
Commuting process between two subpopulations x, y. The cyan and orange arrows indicate the back and forth commuting flows.
In recent decades, the theory of complex networks has been developed as a valuable tool for modeling the structure and dynamics of complex systems. In the study of network epidemiology, networks are often regarded as carriers describing the epidemic spreading such as SARS, H1N1, Ebola, and worm viruses, which are threats to human. In order to control epidemic, we shall understand the dynamics of epidemic, including threshold and steady-state infection. By the development of modern sentinel surveillance systems, high-resolution, temporal-spatial, contact-mobility big data of human are available, which provide efficient information to epidemic researchers. Notably, temporal networks as mediums depict the temporal virus spread between individuals. To characterize the spatial virus spread between different geo-locations, simple network models are generalized with metapopulation framework.
Our research interests on epidemic processes are as follows:
1. Theoretical analysis of the properties, such as epidemic threshold and steady-state infection of epidemic processes
2. Study of immunization strategies
3. Epidemic dynamics on temporal networks
4. Spatial epidemic dynamics on metapopulation networks
Currently, mainstream analytical tools face performance bottlenecks and functional limitations in handling large-scale dynamic network data and complex dynamic simulations. To address these challenges, our team design a high-performance analysis toolkit for epidemic spreading—ViralDynamic [GitHub]. The framework combines several essential functionalities, including temporal network construction, trajectory planning, higher-order dynamics prediction, propagation dynamics simulation, and immunization game evolution analysis. With its flexibility and ease of use, it lowers research barriers and provides strong technical support for researchers and developers.
Key features of ViralDynamic include:
1. TeNet: A temporal network modeling tool for large-scale WiFi data. Allows users to create dynamic networks that represent interactions within a population, simulating disease spread across different types of connections.
2. SubTrack: A trajectory planning tool for massive metro swipe card data. Supports users in obtaining precise individual trajectories during metro trips to analyze mobility patterns.
3. TaHiP: A high-order dynamics prediction tool. Allows users to accurately predict higher-order dynamic with unknown higher-order topology.
4. ε-SIS: A tool for simulating ε-susceptible-infected-susceptible (ε-SIS) dynamics in continuous time.
5. EvoVax: A social perception-based immunization game evolution analysis tool.
The team involved in the ViralDynamic project is detailed in the [About Me], and related publications could be found in the [Related Work].
ViralDynamic Framework
ECG (Electrocardiography) Analysis:
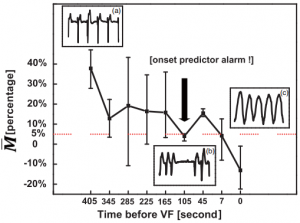
The mean difference M-bar between the percentages of motif C and motif A in associated networks generated from the NSR 5-beat episode to predict ventricular fibrillation before it happens.
Complex networks provides us with a new viewpoint and an effective tool for understanding a complex system from relationship between the elements in global way, and can be a powerful tool for revealing the information embedded in time series. In recent years, the researchers developed many methods to bridge time series dynamics and complex network theory, for example visibility graph, recurrence method. In our research, we analyze the ECG signal based on complex network to detect ventricular arrhythmias.
Synchronization and Coordination of Complex Networking Systems:
Cooperative control of networked multi-agent systems (MASs) has attracted researchers’ wide attention and plentiful investigations on various aspects including consensus, formation, rendezvous, synchronization, swarming, flocking, containment control, and so on. MASs have a broad range of applications in military, aerospace, and industry fields due to the distinguishing feature that a network of simple agents can complete complicated global tasks efficiently by cooperating with neighbors only.
Our research interests on the topic of cooperative control of MASs cover several theoretical hotspots and the extension to realizations, which are listed as follows:
1. Predictive control of networked MASs: predictive consensus or predictive flocking
2. Synchronous/Asynchronous consensus of networked MASs with aperiodic sampled-data
3. Event-triggered predictive control of networked MASs
4. Hardware platform realizations of MASs for further research applications: the agents are ground vehicles or quadcopters
Controllability and Control of Complex Networks:
To gain a complete mastery of the behavior of the dynamical network, one of the most primary problems we need to ensure is whether we could control dynamical network to present any desired performance. By taking full advantage of network topology, nodal dynamics and other favorable factors, by minimizing resource consumption as much as possible, identifying whether network system is controllable and how to control is what we focus on.
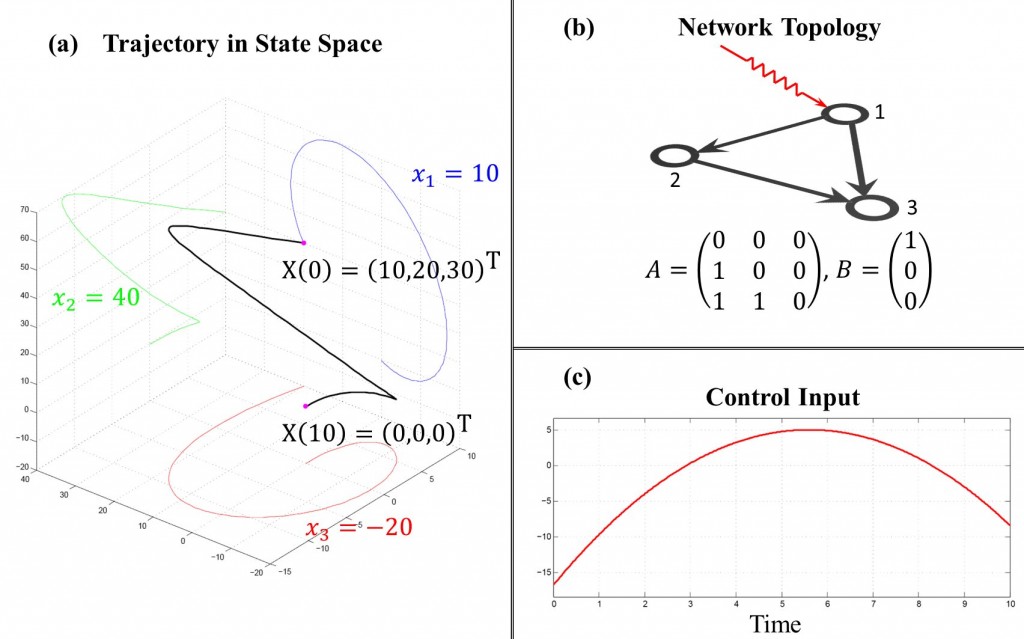
Fig. (a). Toward the network system as shown in (b), we hope that it could be steered from any initial state (e.g., X(0)=(10,20,30)’ ) to any desired final state (e.g., X(0)=(0,0,0)’ ). The red, green, blue curves represent projections on three directions respectively. (c). Control input tells us how to control the network system. But before calculating this, we should first confirm that the network system is controllable. Otherwise, we are likely to do futile actions.